BDFI academic Xenofon Vasilakos, lecturer of AI for Digital Infrastructures with the Dept. of Electrical and Electronic Engineering and a member of Smart Internet Lab at the University of Bristol, discussed the current orchestration systems intelligence gap when devising 6G network services at the IEEE International Conference on Communications this week. Below, he explains a Reinforcement Learning model-based orchestration approach tested with Bristol’s 5G city testbed and a real use case, which tries to address this intelligence gap while aiming at multi-objective optimisation goals. Further, Xenofon explains how this work poses a basis for integrating and supporting sociotechnical aspects in the future, such as fair resource consumption by users and services.
Network softwarisation in the fifth and future sixth generation of wireless networks (5G, 6G) is characterised by significant flexibility and agility as a result of adopting the concepts of Software Defined Networking (SDN) and Network Function Virtualization (NFV). The former have enabled scalable vertical industry services with strict performance requirements that need to be addressed by MANagement and Orchestration (MANO) systems. Nonetheless, today’s state of the art in MANO systems faces fundamental challenges regarding the highly complex problem of optimal user service function placement. MANO systems still lack Machine Learning (ML) intelligence while remaining largely dependent on rule-/heuristic-based solutions focusing exclusively on system-level resources after predefined policies.
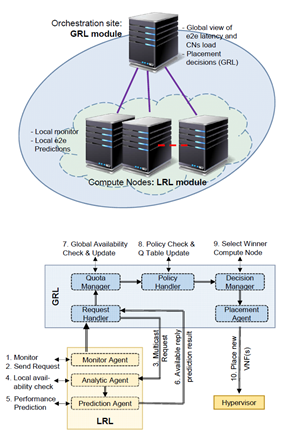
The above approach neglects critical technical aspects such as network dynamics and system-wide service-level performance objectives of both verticals and infrastructure providers as expressed by Key Performance Indices (KPIs) such as service latency or balanced resource utilisation. In addition, it neglects the potential of including Key Value Indicators (KVIs) such as user access fairness to deployed services to avoid user starvation.
To address these gaps, we propose and present our latest work entitled “HELICON: Orchestrating low-latent & load-balanced Virtual Network Functions” to the IEEE International Conference on Communications, in May 2022, Seoul, South Korea (https://icc2022.ieee-icc.org/), during the “QoE And Network Systems” leg of the technical symposium of “Next-Generation Networking and Internet (NGIN)”.
HELICON stands for “Hierarchical rEinforcement LearnIng approach for OrChestratiNg” low-latent and load-balanced services. Though targeting purely technical KPI-based objectives in the current stage, HELICON paves the way for introducing also KVI-based objectives into the MANO equation, thus setting the necessary technical background for supporting socio-technical aspects in current and future 6G MANO operations. In brief, HELICON:
- Poses a novel distributed hierarchical Reinforcement Learning (RL) approach that can serve as a stand-alone online service placement solution as well as a module-based extension for the current state of the art.
- Tackles a computationally/analytically difficult problem (NP-Hard) with a tunable and lightweight Q-Learning scheme that besides KPIs can also support KVIs in the future such as fair access to resources by both users and services. In its current pilot version, HELICON optimises either or both of (i) end-to-end service delay or (ii) load balancing among hosting service nodes.
- Last, we provide a real-life testbed implementation and use case-driven validation, and specifically, practical experimental results upon a realistic 5G Smart City Safety (SCS) use case conducted over a Bristol’s 5G city testbed assuming an e2e application video transcoding service.